You can’t see it
It’s electric boogie woogie, woogie
You gotta feel it
It’s electric boogie woogie, woogie
Ooooh, it’s shocking
It’s electric boogie woogie, woogie
Just as the shininess of generative AI started to lose its polish from the reality of trying to use it, a new buzzword has re-entered the lexicon: agentic.
The term “agentic” refers to AI systems that exhibit autonomy and goal-directed behavior characteristics. These systems go beyond simply generating responses or content based on input—they act as agents capable of making decisions, taking actions, and adapting to achieve specific objectives in dynamic environments.
The concept of autonomous agents is not new. We’re bringing back the classics. In Artificial Intelligence: A Modern Approach (1995), Peter Norvig and Stuart Russell defined an agent as anything that perceives its environment and acts upon it.
The idea that we can automate routine tasks to free up people to do more challenging, more creative tasks is noble and has been a rallying cry of computers and software since their inception. In his essay “As We May Think,” Vannevar Bush imagined a device called the “Memex” to help humans store, organize, and retrieve information efficiently, aiming to reduce mental drudgery and aid creativity.
Computers were first used to automate repetitive, time-consuming industrial tasks, especially in manufacturing. Early pioneers recognized that this freed humans for more complex supervisory roles.
As computers and software became more accessible, researchers explored “expert systems” that were designed to take over repetitive knowledge-based tasks to allow professionals to focus on more challenging problems.
Today, generative AI tools like ChatGPT, Github Copilot, and others are attempting to fully realize this concept by automating tasks like writing, coding, design, and data analysis, allowing humans to concentrate on strategy, creativity, and innovation.
But since the mainstream generative AI boom in 2022, which saw the public availability of ChatGPT and Github Copilot, and the expansion in 2023 and 2024 with more competition in generative AI, the challenges of bringing this technology into the enterprise and driving meaningful value have dampened the early enthusiasm.
That’s not to say that generative AI hasn’t been valuable. Many enterprises report productivity gains1,2 from early use cases like code generation, knowledge management, content generation, and marketing. However, several challenges have made it more difficult to scale and adopt generative AI more broadly.
Hallucinations, where outputs are factually incorrect, fabricated, or nonsensical, despite appearing plausible or confident, introduce risk and distrust into generative AI solutions. Toxic and harmful language, including encouragement of self-harm and suicide3,4, further expose companies and reduce their interest in exposing their customers directly to generative AI output.
Introducing generative AI has also highlighted systemic internal issues. Knowledge management use cases were seen as straightforward, low-risk ways to leverage generative AI. For example, retrieval-augmented generation (RAG) allows users to get context-aware answers by combining AI-generated content with real-time retrieval of relevant information from sources like internal documents and databases.
But what happens when that documentation is missing, outdated, or incorrect? What if the documentation is ambiguous or contradicts itself? What if the documentation is not in a format easily consumed by the RAG system? These generative AI solutions are not a technical solution to poor documentation and data. As the saying goes, “Garbage in. Garbage out.”
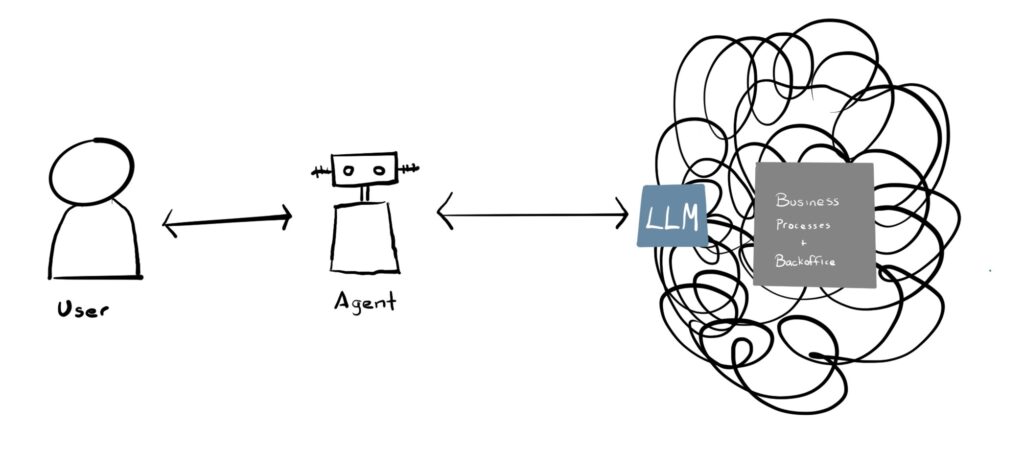
While the challenges above are relevant to most generative AI implementations, one that applies to agentic AI and agents relate to business processes.
Agentic AI relies on well-defined tasks, workflows, goals, and a clear understanding of how processes operate to function effectively. If processes are unclear or undocumented and data is inconsistent, incomplete, or unavailable, the AI may struggle to execute tasks properly or optimize workflows.
Generative AI and agents are not a technical solution to inefficient processes, just as AI isn’t a solution to bad data. Automating an inefficient process with an agent could reinforce and scale those inefficiencies, creating more bottlenecks or errors.
Companies often prefer introducing new technology rather than spending resources updating outdated documentation or optimizing processes. These challenges highlight the risks of skipping those steps, especially when agents can execute transactions automatically and interact directly with customers. The possibilities of financial and reputational damage by a wayward agent are dangerously real.
However, the lure of automation and operational efficiency is strong, and the landscape of offerings in the agentic AI space continues to grow. In 2024, the market size was estimated to be between $5 B and $31 B5,6. By 2032, the market is projected to reach approximately $48.5 B7. Like winning the lottery, that dollar figure is causing companies to forget the struggles of implementing non-agentic generative AI in pursuit of a big payoff through automation. But what opportunities are missed to improve business and customer outcomes without agents (or even AI) while chasing that payoff?
That’s not to say there isn’t a place for agentic AI. Similar to the gains seen from generative AI, the ecosystem of conversational, natural language, multi-model, and adaptive agents can be a powerful tool to solve complex problems and drive value. However, it will take time because work must be done before this value can be fully realized. Paraphrasing a quote, the road to generative AI (and agentic AI) is clearer than ever before, but it’s much longer than we thought.
Recommendations
While we travel that road to the promised land, there are a few areas companies can focus on to prepare for an agentic world:
Invest in documentation management and data quality. If previous AI projects failed due to poor documentation or data, an AI agent will likely have the same fate as its predecessor. Companies may see incremental gains through this effort because it’s likely that poor data and documentation are creating inefficiencies. For example, poor documentation can cause support agents to struggle to find answers, causing longer handling times.
Invest in process optimization. The simpler a process is, the more likely it can be automated. I’ve found that companies want to keep their complex processes, which humans often find challenging to navigate, and think that automating them is a faster path to efficiency gains. The reality, however, is that complex processes have a long tail of edge cases that cause automation to break down, require extensive troubleshooting and tuning, and cancel out value.
Simplify architectures and APIs. One aspect of autonomy for agentic AI is access to tools and functions that the agent can execute to act. An agent cannot effectively utilize complex APIs that wrap multiple functions and are not well-instrumented.
Focus on risk mitigation. As mentioned above, generative AI and agentic AI introduce risks, including hallucinations, toxic and harmful language, and a lack of oversight and controls. If the best time to plant a tree is 20 years ago, the best time to implement guardrails and controls is before introducing agents. As business processes are reviewed, optimized, and documented, attention should be paid to identifying and securing vulnerable points.
Identify small use cases with low risk and high value. It can be tempting to throw agentic AI at the biggest or most expensive problem to maximize the return on investment. However, starting with complex, high-stakes processes increases the likelihood of errors, inefficiencies, and stakeholder resistance. Instead, focus on areas where agentic AI can deliver quick wins. This approach allows teams to refine their understanding of the technology, build trust, and develop best practices before scaling to more critical or complex use cases.
Consider non-agentic and non-AI solutions. Of all the recommendations, this one will likely generate the most resistance as companies are pushing towards the promise of generative AI and agentic AI solving all problems. Improving customer service or reducing call volume through better internal documentation or website search won’t generate enough buzz to show up in a news feed. There is so much pressure to find problems that can be solved with generative AI, forcing a solution-first, technology-first mindset. Ultimately, it should never be about the technology. It should be about the outcomes. It should be about improving our customers’ and employees’ lives and experiences and the value we bring to the business. Start with a problem or pain point and work backward. Consider all possible solutions, and choose the one most likely to succeed, even if it doesn’t feed the hype.
Conclusion
While the allure of agentic AI is undeniable, achieving its promised potential requires deliberate preparation, thoughtful execution, and a focus on foundational improvements.
Companies must resist the urge to chase the hype and prioritize efforts that enhance data quality, streamline processes, and establish robust risk mitigation strategies.
Starting with low-risk, high-value use cases can build momentum, trust, and a clear path to scalable adoption. At the same time, leaders should remain open to non-agentic and non-AI solutions that more effectively and sustainably address pain points.
Ultimately, the goal should not be to implement the latest technology for its own sake but to deliver meaningful outcomes that enhance customer experiences, empower employees, and drive long-term business value.
The journey toward agentic AI may be longer and more complex than we thought, but with the right approach, we can significantly increase the likelihood of realizing its full value.
You can’t see it. You gotta feel it. Ooooh, it’s shocking. It’s agentic.
Footnotes
- https://cloud.google.com/resources/roi-of-generative-ai ↩︎
- https://www.wsj.com/articles/its-time-for-ai-to-start-making-money-for-businesses-can-it-b476c754 ↩︎
- https://gemini.google.com/share/6d141b742a13 ↩︎
- https://apnews.com/article/chatbot-ai-lawsuit-suicide-teen-artificial-intelligence-9d48adc572100822fdbc3c90d1456bd0 ↩︎
- https://www.emergenresearch.com/industry-report/agentic-artificial-intelligence-market ↩︎
- The wide range in the 2024 figure is likely due to differing methodologies or market definitions. ↩︎
- https://dataintelo.com/report/agentic-ai-market ↩︎